Imagine you had the ability to call upon thousands of years of human experience, writing, art and media from a single prompt. That is the power of generative AI, a technology that has revolutionized various industries and is poised to be one of the most important tools in human innovation and productivity in the coming years.
In this article, we will explore what generative AI is as well as its applications and potential implications, providing a comprehensive overview of this extremely compelling technology.
What is generative AI?
Generative AI is a form of artificial intelligence that uses deep-learning models to produce new content such as text, images, speech, code and music. It does this by learning from existing artifacts, allowing it to generate new, high-quality content that is reflective of the data it was trained on, but doesn’t repeat it.
For example, Midjourney creates brand new images by analyzing patterns in a vast dataset of existing visuals and generating unique artwork that follows those learned patterns. The process consists of a few key components.
Content creation
By leveraging advanced algorithms, generative AI can generate high-quality text, images, audio, video and product designs tailored to specific audiences and contexts. This automation not only speeds up the content production process but also enables scalability and personalization, quickly meeting end-user needs with a high degree of accuracy.
Example: Generative AI can generate personalized marketing emails that are unique to individual customer preferences. This automation speeds up the content production process and enables companies to scale tailored communications that meet the specific needs of their audience.
Machine learning
Machine learning (ML) uses sophisticated algorithms to analyze vast amounts of data, identify patterns and make informed decisions. Through continuous learning from new data inputs, these algorithms can improve their accuracy and predictive capabilities over time.
Example: Machine learning algorithms can optimize logistics and supply chain management by analyzing historical data to predict demand and manage inventory levels. As they continuously learn from new data inputs, their accuracy and predictive capabilities improve, ensuring ever-increasing supply chain efficiencies.
Natural language processing
Natural language processing (NLP) focuses on the interaction between computers and human language. It enables machines to comprehend, interpret and respond to text or speech inputs in a way that is both meaningful and contextually relevant.
NLP powers a wide range of applications, including chatbots, sentiment analysis and automated translation—enhancing the ways in which businesses engage with customers and analyze textual data. This ability has been instrumental to a number of case studies that we will see shortly below.
Example: NLP can play a major role in healthcare by providing comprehensive and consistent documentation of patient medical records. A study led by the University of Pennsylvania demonstrated NLP-generated data allowed for the creation of new quality metrics, ensuring care better aligned with patient goals and values as well as streamlined administrative tasks while enhancing overall care quality.
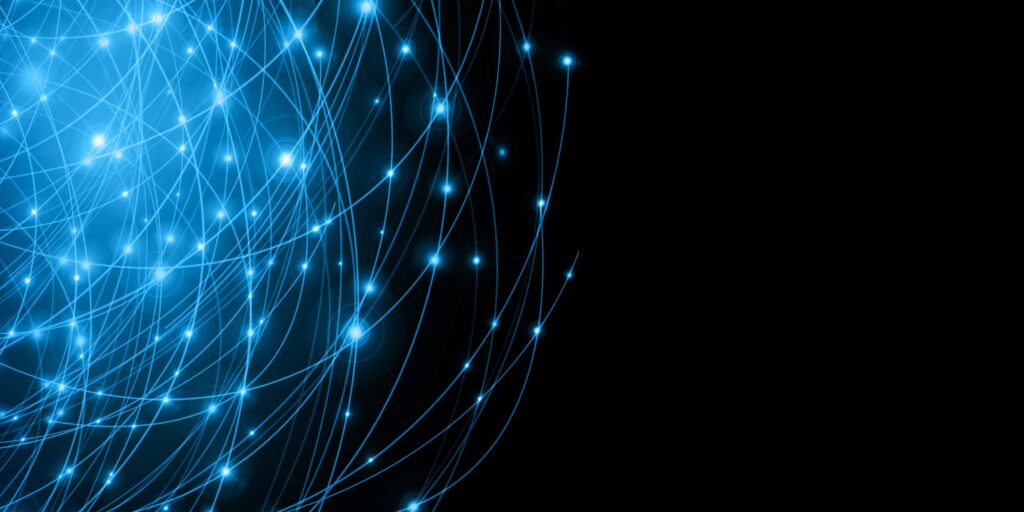
Our AI Strategy for Creating Dataminr-unique High Performance LLMs and Foundation Models
To realize Dataminr’s expansive vision, our AI strategy has been to create a broad set of Dataminr-unique, customized foundation models and LLMs.
Learn MoreAdaptive learning
Adaptive learning refines the AI’s learning process based on its performance and accuracy as it is exposed to more data and user interactions. This dynamic improvement process allows AI to become more efficient and effective, tailoring its functions to better meet user needs.
Example: Adaptive learning allows AI in e-commerce to refine its product recommendations by analyzing user interactions and purchase behavior, becoming more precise over time. This continuous improvement process enables the AI to better anticipate user preferences and enhance the shopping experience.
Harness high-quality data
The quality and volume of data directly influence the performance of generative AI, making data collection and management critical components of the models. Leveraging large datasets enables AI to provide insights and solutions that are both precise and impactful, driving informed decision making and innovation.
Example: As with natural language processing, data-driven content creation can be used in healthcare. Leveraging large datasets allows generative AI to predict disease outbreaks with high precision by analyzing vast amounts of medical data. This robust data collection and management enable more informed decision making in patient care and treatment strategies.
History and evolution of generative AI
Nothing operates in a vacuum and the development of AI from where it was to where it is today is no different. Machines that served as precursors to modern day generative AI focused on rule-based systems and data analysis, paving the way for the tech to be able to create original content.
The 1956 Dartmouth Conference is often hailed as the birth of AI as an academic subject, bringing together a diverse group of minds to set the foundation for the burgeoning field of artificial intelligence. This seminal event laid the groundwork for future developments, marking the start of structured exploration into deep learning and neural networks.
What followed were years of AI advancements that, to this day, continue to break barriers. Here we outline the evolution of AI, from the mid-80s to present day.
Evolution of AI: Mid-80s to Present Day
- 1986: Backpropagation Algorithm. The backpropagation algorithm, popularized by Geoffrey Hinton, David Rumelhart, and Ronald Williams, revolutionized neural networks by enabling the practical training of multi-layered networks. This breakthrough allowed for the effective learning of complex patterns in data, laying the foundation for modern deep learning techniques.
- 1997: Deep Blue Defeats Garry Kasparov. In 1997, IBM’s Deep Blue, a chess-playing computer, defeated world chess champion Garry Kasparov. This event demonstrated AI’s capability to perform tasks requiring strategic thinking and complex decision-making, marking a significant milestone in AI development.
- 2011: IBM Watson Wins Jeopardy! IBM Watson, a question-answering computer system, won the game show Jeopardy! against two of the show’s greatest champions, Ken Jennings and Brad Rutter. This achievement showcased the advancements in natural language processing and the ability of AI to understand and respond to complex queries.
- 2012: AlexNet. AlexNet, a deep convolutional neural network architecture, was specifically designed for image classification tasks, pioneering the use of deep learning in computer vision and paving the way for advancements in image recognition, object detection and related fields. It was critical in revitalizing interest in neural networks and their potential to revolutionize AI research and applications across various domains.
- 2014: Generative adversarial network. Introduced by Ian Goodfellow in 2014, the generative adversarial network (GAN) was a significant breakthrough in the field of AI because it managed to generate realistic images and other data. This breakthrough highlighted the evolving capabilities of deep learning and neural networks in creating high-fidelity synthetic data.
- 2015: AlphaGo Defeats Lee Sedol. Google DeepMind’s AlphaGo defeated world champion Go player Lee Sedol. This event was significant because Go is a complex game with more possible moves than atoms in the universe, showcasing AI’s ability to handle extremely complex tasks and strategic thinking.
- 2020: GPT-3. OpenAI’s release of GPT-3, commonly known as ChatGPT, marked a significant leap in generative AI capabilities, showcasing the potential of large-scale language models (LLMs). GPT-3’s ability to generate coherent text that could be largely indistinguishable from human output underscored the advancements in deep learning and neural networks, further propelling the evolution of AI.
- 2023: GPT-4. OpenAI’s release of GPT-4, demonstrated even more sophisticated understanding and generation of human language, solidifying the role of AI in various applications from natural language processing to creative writing.
Current state of generative AI
Generative AI’s usage spans the consumer, public and private sectors, demonstrating its versatility and impact. While we are still in the early days of applicability, various organizations are experimenting with the technology for a myriad of purposes. In this section, we’ll cover AI capabilities across government and private sector use cases.
Public sector security
Many governments have begun using generative AI for two primary reasons:
- Leveling up strategic planning: Vast amounts of data can be analyzed to identify emerging threats, assess geopolitical risks and optimize resource allocation for military operations. Leveraging AI algorithms for data analysis can help the tech make more informed decisions.
- Enhancing cybersecurity measures: AI-powered systems can be used for network monitoring, anomaly detection and threat intelligence analysis to proactively respond to cyber attacks. Generative AI’s adaptive learning component allows organizations’ to continuously adapt to evolving threats and vulnerabilities.
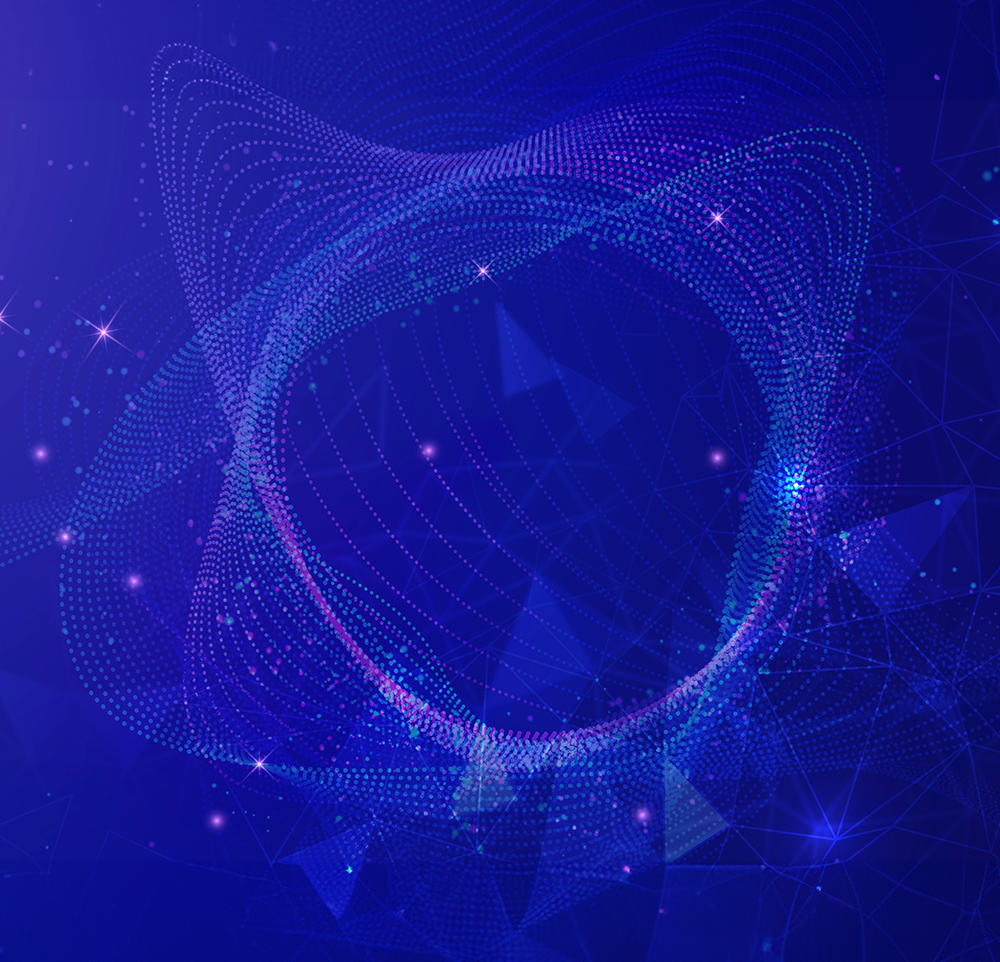
GenAI In State and Local Government: Adoption Challenges and Tips
The adoption of generative AI in the public sector has been slow. Read on to learn key adoption challenges and recommendations.
Read NowPrivate sector efficiency
In the private sector, AI is being leveraged for distinct competitive advantages. More specifically, some organizations have begun to integrate it into every department. As a result, many have noticed significantly improved efficiencies with reduced customer and employee support workloads in addition to raising productivity in software development teams.
While media headlines extol AI job replacement scenarios, in many private sector use cases, AI is primarily enhancing or augmenting the human component—not replacing it. Ongoing investments in the technology is expected to make things more efficient and better for all stakeholders involved.
Challenges and concerns
As businesses increasingly adopt AI technologies, several considerations surrounding misinformation have become paramount. The potential for AI to spread false or misleading information can have severe consequences for both businesses and society at large. Bias embedded within the training data itself can perpetuate misinformation if not carefully monitored and corrected.
Additionally, generative AI is known to sometimes hallucinate data, producing outputs that seem plausible but are factually incorrect or entirely fabricated. This occurs due to the model’s reliance on patterns found in its training data, which can sometimes lead it to generate information that isn’t grounded in reality.
Therefore, companies must implement robust verification mechanisms and ensure transparency in their AI processes to mitigate these risks. This kind of vigilance is crucial for maintaining public trust and safeguarding the integrity of business operations.
Regulatory responses to AI’s rapid advancement have sparked ongoing debates, especially concerning the time lag between technological progress and the slow pace of legal frameworks.
Security risks have also escalated with the advent of sophisticated AI tools, ushering in a new era of hacking and cyber threats. These risks include the potential for AI-driven attacks that are more complex and harder to detect than traditional methods. Hackers can automate and scale their attacks, targeting vulnerabilities at unprecedented speeds.
Businesses must enhance their cybersecurity frameworks to counter these new threats, incorporating advanced defenses such as AI-based intrusion detection systems and real-time threat analysis. Staying ahead of these risks is essential for protecting sensitive data and maintaining operational resilience.
Regulatory responses to AI’s rapid advancement have sparked ongoing debates, especially concerning the time lag between technological progress and the slow pace of legal frameworks. AI regulation aims to address concerns about AI ethics, biases and security risks, but the process of enacting and enforcing laws around the world is often lengthy and complex.
As AI technologies evolve swiftly, the regulatory landscape struggles to keep up, creating gaps that can be exploited. Businesses must navigate this uncertain environment by proactively adhering to best practices and engaging with policymakers to shape effective regulations. This proactive approach will help companies harness the benefits of AI while mitigating ethical and security concerns.
The persistent use and training of personalized AI overtime will result in businesses being able to develop customized experiences for their clients.
All this is to say that businesses must approach generative AI with a balanced and forward-thinking strategy. By prioritizing ethics and addressing biases, companies can take advantage of automation while building trustworthy systems that minimize misinformation at the same time.
Enhancing cybersecurity measures to counter AI-driven threats is critical for protecting business assets and customer data. Finally, actively participating in the regulatory discourse will help shape a future where the technology can thrive responsibly and securely, aligning with broader societal expectations.
Benefits and opportunities for generative AI
The adoption of generative AI has led to remarkable efficiency and productivity improvements for operations across almost each and every business sector. By leveraging AI efficiency, businesses can automate complex processes and streamline operations, significantly reducing the time and resources required to complete a variety of tasks.
Traditionally time-consuming tasks that required several layers of human oversight can now be automated by AI. Generating reports, creating and designing marketing materials, and even drafting legal documents can be developed through a single or series of prompts. This level of productivity, never before witnessed in human history, can vastly accelerate workflows as well as consistency of output, allowing individuals to focus more on strategic and creative initiatives.
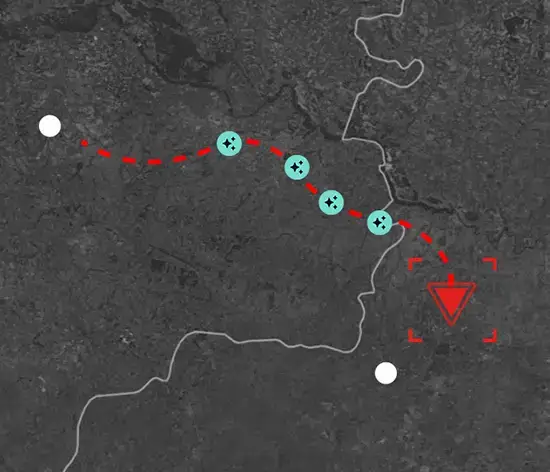
The Power of ReGenAI
Dataminr’s ReGenAI—a new form of generative AI that automatically regenerates textual descriptions of events as they unfold—gives customers a rapid understanding of multidimensional events in real time.
Watch VideoAdditionally, this automation gives human creators more time to focus on nuanced creative processes that can be used to make AI even more sophisticated. The persistent use and training of personalized AI overtime will result in businesses being able to develop customized experiences for their clients. Examples include:
- Targeted marketing campaigns
- Bespoke product recommendations
- Unique content creation
- Enhanced enterprise search capabilities
- Streamlined IT support desk operations
- Accelerated code development
- Responsive customer support
- Dynamic financial forecasting
- Predictive maintenance in manufacturing
- Advanced medical diagnostics
This tailored approach will likely exceed customer expectations, driving higher engagement and loyalty. For example, in the fashion industry, AI can generate new designs based on current trends and consumer preferences, leading to a more dynamic and responsive product development process.
The competitive edge that generative AI offers is becoming hard to ignore. Companies leveraging AI productivity in their operations are poised to outstrip their competitors, thanks to boosted efficiency, productivity, and innovation. AI-driven insights empower more informed decision making and quicker responses to market dynamics. Those who resist adopting these technologies risk lagging behind with outdated, manual processes.
Generative AI also enables rapid adaptation to consumer demands, delivering agility and personalization crucial in today’s fast-paced market. In essence, strategically implementing generative AI can establish a business as a leader, ensuring a sustainable competitive advantage.
Future outlook
The future of generative AI is set to bring transformative changes across all businesses and governments. By 2027, more than 50% of generative AI models organizations use will be specific to either an industry or a business function, up from 1% in 2023. Within the public sector, 25% of governments already have or plan to deploy generative AI; an additional 25% are planning to deploy it in the next 24 months.
This means that generative AI models will take on more domain-specific use cases that are more accurate and lower the risk of hallucinations found in general purpose models.
Finally, no discussion around “what is generative AI” would be complete without at least touching on the concept of artificial general intelligence (AGI). AGI aims to mimic human intelligence across a wide range of tasks, demonstrating complex reasoning, problem solving and adaptability. It can be likened to a rational system capable of performing any intellectual task a human can—crucially the ability to make decisions on incomplete information and learning new skills independently.
The future may see gradual advancements in hyper-specific applications of generative AI paving the way for autonomous AGI systems. However, achieving AGI remains a complex challenge, requiring breakthroughs in understanding and replicating human cognitive processes in addition to the moral and ethical considerations. The continuous evolution of AI models and the pursuit of AGI will shape the landscape of technology, driving progress and opening new frontiers in various domains.
Generative AI is at the forefront of human ingenuity. As it revolutionizes the business operations of numerous industries, there will be an exponential number of new opportunities for progress across all aspects of society. The advanced capabilities in content creation, machine learning, natural language processing, adaptive learning and data-driven decision making are critical ingredients, but the human spirit of innovation will drive just how far we can go.
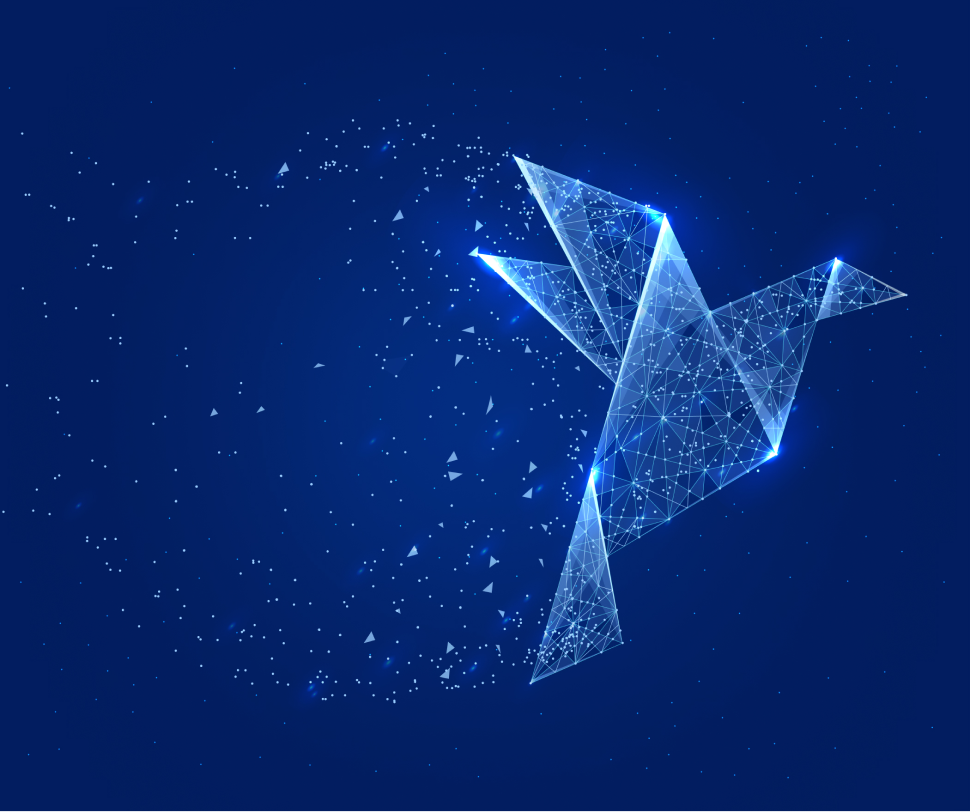
Dataminr’s AI Platform
Dataminr’s AI platform is at the forefront of innovation in predictive, generative and regenerative AI. Get a first-hand look to see how it helps organizations like yours strengthen organizational resilience in an increasingly unpredictable world.
Request a Demo